IT Pro Today. Irritated cats supplied first reinforcement-learning data, Future Infinitive explains
- Lyle Neff
- Apr 7, 2020
- 2 min read
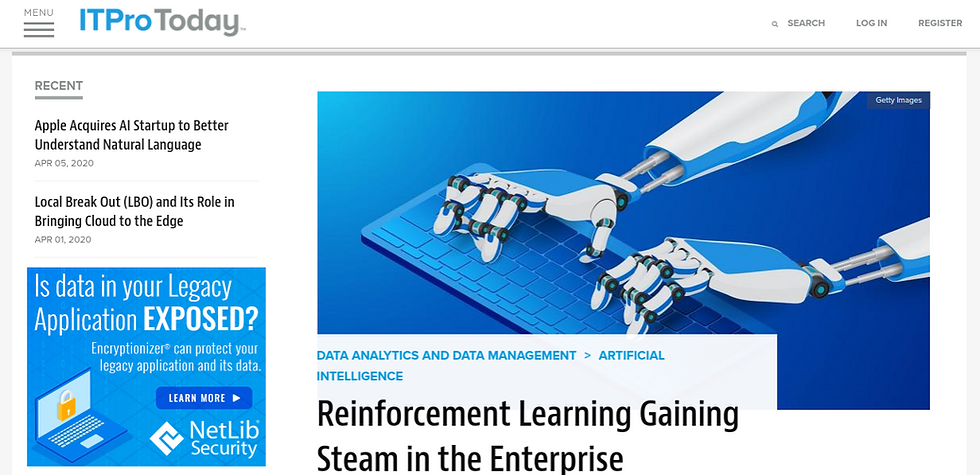
Two schools of thought contend, and sometimes co-operate, in the race to develop valuable AI enterprise tools.
The broad division is between deep learning techniques, which glean information from one dataset and apply it to another (think facial recognition) -- and reinforcement learning, where the program uses a continuous feedback loop to adjust its response and maximize a “reward” (think AIs that play video games).
The border between the two approaches is hazy, but deep learning is more about data. Reinforcement learning is more about rules, and is starting to seem the more promising approach, author Terri Coles reports here.
It’s funny to think, says her interview subject Vaclav Vincalek, a partner in Future Infinitive, that it all began over a century ago with a whiff of fish.
Read Vaclav Vincalek on Edward Thorndike and the first reinforcement-learning data.
Early behaviourists noted that cats trying to navigate their way out of a puzzle box learned to do so faster, and retained that skill longer, when tempted with a piece of fish. Generations of thinkers built on this key insight about positive consequences, Vincalek says, resulting in powerful RL applications for situations
“‘...where the environment is known but the analytical solution is not.’
“Some of the areas where reinforcement learning makes sense are clear if you think about what improves with practice when done by humans — for example, driving. Reinforcement learning has been used in AI driving simulations where virtual cars complete a course over and over and over.
“Theoretically, that approach could be behind the software powering real-world autonomous vehicles following set routes. Mobileye, Google and Uber have said they are testing reinforcement learning for their vehicles.
“Reinforcement learning helps companies see which action yields the higher reward over the longest period, Vincalek said.”
Reinforcement-learning data usage sparks a wide array of applications
Machine learning in general has found its greatest success in mimicking the way humans learn, rather than the way we behave. Reinforcement learning in particular seems to correlate to certain truths about rewards and punishments in human nature. But mimicry is not replication, and the computing technique has some evident limitations.
For example, machine learning is “suited to controlled environments,” as IT Pro says -- “but some environments are uncontrollable and all have unexpected events — a child who runs across the road, for example, or a global pandemic that roils stock markets.”
It’s best to think of reinforcement learning, at this point in its development, as an aid to human intuition, and not a potential replacement. The most currently-useful RL applications, like teaching helpfulness to robotic dogs, follow this supplementary logic.
A pragmatic approach will make the best use of reinforcement-learning data
The technique’s potential remains high. But pragmatism requires that we note there’s no actual learning going on in reinforcement learning. As the father of the field, Edward Thorndike himself, said over a century ago: “There is no reasoning, no process of inference or comparison; there is no thinking about things, no putting two and two together; there are no ideas — the animal does not think of the box or of the food or of the act he is to perform.” Neither does the computer.